AI-powered facial recognition technology is changing the way employment background checks are conducted. This advanced tool uses complex algorithms to analyze and compare facial features with a wide range of data sources, making it easy to identify individuals quickly. Employers are increasingly using this technology to make their screening processes more accurate and efficient.
The use of AI in employment screening evokes several essential ethical issues:
- Privacy Concerns: The collection and storage of biometric data raise questions about how you protect personal information.
- Consent: How much candidates know about and agree to their facial data used during background checks.
- Bias and Discrimination: Possible biases programmed into AI algorithms that could result in unfair treatment based on race, gender, or ethnicity.
These concerns show the importance of finding a fair balance that allows for the advantages of using AI-powered facial recognition in employment background checks while protecting individual rights.
Understanding AI-Powered Facial Recognition for Employment Background Screening
AI can be a powerful tool for identifying and screening job applicants. Instead of manually reviewing documents and records, this digital solution uses complex algorithms to analyze facial features in images or videos.
Primary Functions of AI in Background Checks:
Here are the main ways AI is used in background checks:
- Identification: Comparing an applicant’s photo with a database of images to confirm their identity.
- Screening: Checking visual data against criminal databases to identify potential risks.
- Automation: Enhancing the verification process by reducing the need for manual data entry and review.
Surveillance cameras are crucial in making this technology work effectively. They provide clear and detailed visual data that these AI systems rely on. By combining AI-powered facial recognition with surveillance camera data, employers can access tools that enhance accuracy and dependability in their background check procedures.
The Case of the NY Student Protests: Examining the Ethical Implications
The NY student protests provide a critical case study for scrutinizing the ethics of facial recognition software in employment settings. Triggered by heightened surveillance measures, students rallied against what they perceived as invasive oversight, sparking a broader discourse on privacy rights and technology’s reach.
Ethical Concerns Raised:
- Privacy Intrusion: Deploying facial recognition technology for background checks may impact individual privacy, capturing biometric data without explicit consent.
- Freedom of Expression: As seen during the NY student protests, the use of facial recognition could deter individuals from participating in lawful demonstrations, fearing repercussions like blacklisting or employment discrimination.
Students can face misdemeanor charges from disorderly conduct and trespassing to resisting arrest and obstruction of governmental administration.
While misdemeanor charges are generally considered less severe than felonies, they can still have profound implications for individuals involved in protests. Convictions may result in fines, probation, community service, or incarceration. Moreover, having a criminal record can impact future employment prospects and potentially lead to discrimination or blacklisting.
Mitigating Bias and Ensuring Fairness in AI Background Checks
Employers must actively work towards identifying and rectifying any biases that could unfairly impact job candidates. Here are some essential approaches to consider:
Diverse Dataset Representation
A diverse dataset is essential for training AI models to make fair decisions. Here’s what employers can do:
- Collect Comprehensive Data: Gather information from various individuals, including different age groups, genders, ethnicities, and other relevant characteristics.
- Regularly Update the Dataset: Make sure the dataset is continuously refreshed to reflect changes in the workforce composition over time. This renewal helps prevent outdated biases from influencing the results.
Algorithmic Audits
Auditing the AI algorithms used in background checks can provide valuable insights into their fairness. Here’s how it can be done:
- Seek Third-party Reviews: Involve external experts who can independently assess the algorithms and identify potential biases.
- Be Transparent About Methodology: Share information about how the AI system works, including the criteria used for decision-making. This transparency promotes accountability and allows for scrutiny.
- Use Feedback to Improve: Actively incorporate audit feedback to refine the algorithms and reduce biases over time.
Human Oversight and Intervention
While AI technology significantly automates background checks, human involvement remains crucial for fairness. Here’s what employers should prioritize:
- Conduct Thorough Reviews: Have trained personnel carefully examine the results generated by the AI system to catch any errors or inconsistencies.
- Give Final Decision-making Authority to HR Professionals: Empower human resources (HR) staff to make the ultimate hiring decisions based on their expertise and judgment rather than relying solely on AI recommendations.
- Provide Training on Bias Awareness: Educate HR and hiring managers about the potential biases that can arise from using AI in recruitment processes. It helps them make more conscious and informed choices.
By implementing these strategies into their hiring procedures, companies can create a more equitable recruitment environment where every candidate has an equal chance of success, regardless of their background or affiliations.
Building Trustworthy and Ethical AI Systems for Hiring
Developing trustworthy and ethical AI systems is vitally important in the employment sector, where they play a crucial role in making hiring decisions. Giving importance to transparency and explainability aligns with more comprehensive regulatory requirements, such as the General Data Protection Regulation’s (GDPR’s) right to explanation, which states that individuals should be able to understand and question automated decisions.
Critical Aspects of Trustworthy AI in Hiring:
- Transparency
Companies must provide clear documentation on how their AI systems operate. This information includes the data sources used, the decision-making criteria, and the logic behind algorithmic outputs.
- Explainability
AI should not be a mysterious process. Candidates should understand how their data is analyzed and how decisions are made. An explainable AI system allows for better understanding and trust among all parties involved.
- GDPR Compliance
Under GDPR, individuals have rights when it comes to their personal data. AI systems must be designed to uphold these rights by ensuring data protection, accuracy, and the ability to challenge automated decisions.
By incorporating these principles into AI systems for employment background checks, companies show responsibility and create an environment where technology is used ethically. The aim is to utilize innovative tools without compromising individual rights or societal values.
Get instant updates on Background Screening 101
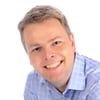
About Michael Klazema The author
Michael Klazema is the lead author and editor for Dallas-based backgroundchecks.com with a focus on human resource and employment screening developments